AI-powered cancer diagnosis in resource limited settings
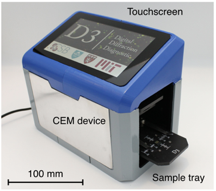
A major global health challenge is the rising incidence of cancers in under-developed regions. Due to limited resources and specialists, correct diagnoses are often delayed by weeks to months and therapeutic windows are missed. The research team in the MGH Center for Systems Biology led by Dr. Ralph Weissleder has now developed a new point-of-care technology for prompt cancer diagnosis. The technology integrates computational optics with artificial intelligence (AI) to facilitate rapid and robust analysis of clinical samples obtained by fine-needle aspiration.
The technology, named CEM (contrast-enhanced micrography), integrates a high-resolution semiconductor imaging sensor, on-chip staining protocols, and deep learning algorithms, to enable rapid lymphoma detection and classification. Unlike conventional microscopy which has an inherent trade-off between resolution and throughput, the CEM system can produce high-resolution images in a large field-of-view (25 mm2). The AI engine based on deep-learning then recognize and analyzes >10,000 individual cells within 12 seconds. Such digital imaging and processing offers fully automated readouts obviating the need for highly skilled health workers or sophisticated analytical techniques.
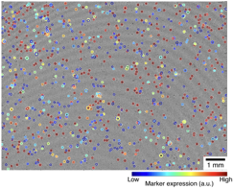
The deep learning algorithm identified B-cells and their locations. The images of found cells were further processed to measure the nuclear size and marker expression, depicted by different-sized circles and their colors. The field of view (FOV) was ~ 25 mm^2^, about 100 times larger than that of a 20× bright-field microscope.
In the current clinical study involving 40 patients, the investigators focused on B-cell lymphomas and the clinical need to reliably differentiate aggressive from indolent subtypes. B-cells obtained from fine-needle aspirate samples were selectively captured on a disposable cartridge and immunostained on-chip against kappa/lambda light chains or Ki67. Based on such molecular information along with size measurements, the AI-driven algorithm 1) identified B-cell lymphoma with 91% sensitivity and 100% specificity and 2) triaged aggressive subtypes with 86% accuracy. In the long term, next generation CEM technology will help catalogue detailed molecular analyses of lymphomas and other highly prevalent malignancies in low and middle income countries.
Article
Im H, Pathania D, McFarland PJ, Sohani AR, Degani I, Allen M, Coble M, Kilcoyne A, Hong S, Rohrer L, Abramson JS, Dryden-Peterson S, Fexon L, Pivovarov M, Chabner B, Lee H, Castro CM, Weissleder R
Design and clinical validation of a point-of-care device for the diagnosis of lymphoma via contrast- enhanced microholography and machine learning
Nature Biomedical Engineering. 2018; DOI: 10.1038/s41551-018-0265-3
Press Coverage
Holographic diagnosis of lymphoma – Nature Biomedical Engineering News & Views
AI-powered cancer diagnoses for resource limited settings – Nature Bioengineering blog